Extensible Data Quality Observability
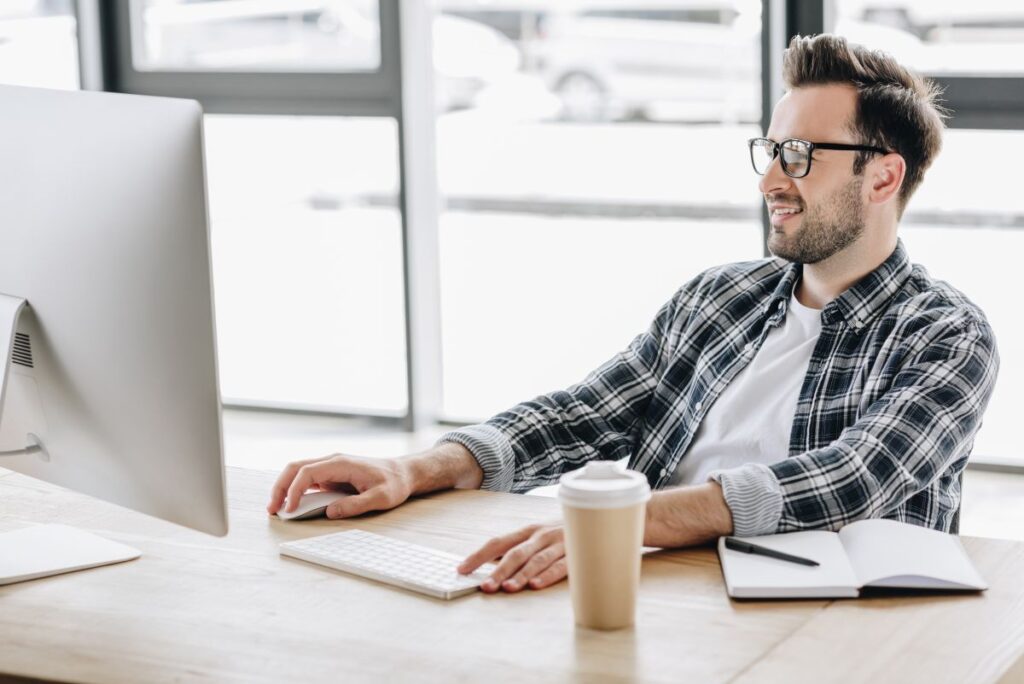
DQO is a methodology used to build data quality into every stage of data processing increase confidence in the final product. The goal of DQO is to ensure that data quality issues are identified and corrected as early as possible in the data processing cycle.
Many organizations struggle with maintaining high data quality because they do not have visibility into their data. Without visibility, it is difficult to identify and correct errors. DQO addresses this problem by providing a framework for tracking the data quality at each stage of processing. By tracking the data quality, organizations can be confident that they are producing high-quality data products.
DQO consists of four steps:
1. Define the scope of the project.
2. Identify the objectives of the project.
3. Collect data about the project.
4. Analyze the collected data.
These four steps will help you understand what needs to be done to improve your data quality.
Defining the scope of the project will help you understand what kind of data you need to collect and how much data you need to collect. Identifying the objectives of the project will help you prioritize which aspects of the project are most important. Collecting data about the project will help you understand what is currently happening and how it can be improved. Analyzing the collected data will help you identify trends and areas that need improvement.
DQO is an iterative process, meaning that it should be repeated as often as necessary to maintain high data quality. By tracking the data quality at each stage of processing, organizations can be confident that they are producing high-quality products.
Extensible Data Quality Observability (DQO) is a methodology used to build data quality into every stage of data processing in order to increase confidence in final products. DQO consists of four steps: defining the scope of the project, identifying objectives, collecting data, and analyzing collected data. DQO is an iterative process, meaning that it should be repeated as often as necessary to maintain highdata quality . By tracking thedata quality at each stage ofprocessing , organizations can be confident that they are producing high-quality products . https://dqo.ai/